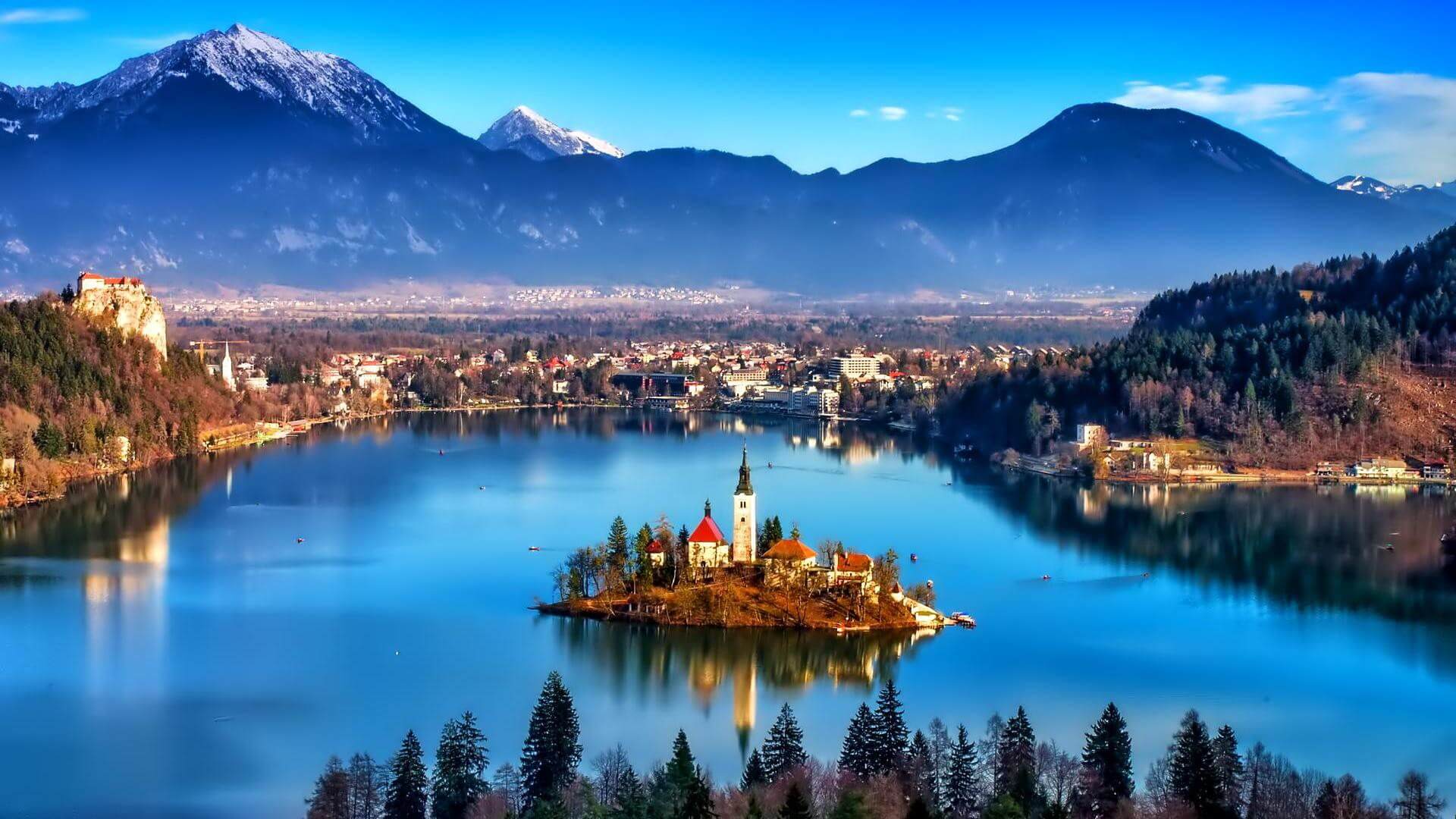
- What is a Data Lake?
- Why are Data lakes central to the modern data architecture?
- What does a data lake promise?
- What are the challenges?
- Why are Data lakes central to the modern data architecture?
- Summary
A data lake is a storage repository that holds a vast amount of raw data in its native format, including structured, semi-structured, and unstructured data. The data structure and requirements are not defined until the data is needed.
- Increased agility
- Data democratization
- Increased innovation speed
- New quicker Insights with advanced analytics
- Improved Scalability
- Self Service
- Get better data quality
- Support all data formats
- Store all types of data in its raw format
- create refined, standardized, trusted datasets for various use cases
- Store data for longer periods of time to enable historical analysis
- Query and access the data using a variety of methods
- Manage streaming and batch data in a converged platform
- Provide shorter time-to-insight with proper data management and governance.
There are three main challenges I see while building a data lake:
- Building - rate of change, skills gap, complexity
- Managing - Ingestion, lack of visibility and privacy/compliance
- Delivering - Quality issues, Reliance on IT, reusability
By understanding the inputs, outputs, processes, and policies within each zone, you can take your implementation further, evaluating a holistic approach and rethinking the possibilities when it comes to build vs buy for the future of your data lake management.
The four zones of physical storage in a data lake architecture:
- Transient landing zone
- Raw zone - When designing the raw data zone, focus on the optimal write performance
- Trusted zone
- Refined zone -When designing the curated data zone, focus on the ease of data discovery and optimal data retrieval
The key considerations when evaluating technologies for cloud-based data lake storage are the following principles and requirements:
- Scalability
- Durability
- Support for unstructured, semi-structured and structured data
- Independence from fixed schema
- Separation from compute resources
Given the requirements, object-based stores have become the de facto choice for core data lake storage. AWS, Google and Azure all offer object storage technologies. A data lake will typically have additional “layers” on top of the core storage. This allows the retention of the raw data as essentially immutable, while the additional layers will usually have some structure added to them in order to assist in effective data consumption such as reporting and analysis. A specific example of this would be the addition of a layer defined by a Hive metastore. In a layer such as this, the files in the object store are partitioned into “directories” and files clustered by Hive are arranged within to enhance access patterns.
Best practices for storage:
-
Partition your data
- Partitioning divides your table into parts and keeps the related data together based on column values such as date, country, region, etc. Partitions act as virtual columns. You define them at table creation, and they can help reduce the amount of data scanned per query, thereby improving performance. You can restrict the amount of data scanned by a query by specifying filters based on the partition.
-
Bucket your data
- Another way to partition your data is to bucket the data within a single partition. With bucketing, you can specify one or more columns containing rows that you want to group together, and put those rows into multiple buckets. This allows you to query only the bucket that you need to read when the bucketed columns value is specified, which can dramatically reduce the number of rows of data to read.
-
Use Compression
- Compressing your data can speed up your queries significantly, as long as the files are either of an optimal size (see the next section), or the files are splittable. The smaller data sizes reduce network traffic from Amazon S3 to Athena.
-
Optimize file sizes
- Queries run more efficiently when reading data can be parallelized and when blocks of data can be read sequentially. Ensuring that your file formats are splittable helps with parallelism regardless of how large your files may be.
-
Optimize columnar data store generation
- Apache Parquet and Apache ORC are popular columnar data stores. They provide features that store data efficiently by employing column-wise compression, different encoding, compression based on data type, and predicate pushdown. They are also splittable. Generally, better compression ratios or skipping blocks of data means reading fewer bytes from Amazon S3, leading to better query performance.
-
Optimize ORDER BY
- The ORDER BY clause returns the results of a query in sort order. To do the sort, Presto must send all rows of data to a single worker and then sort them. This could cause memory pressure on Presto, which could cause the query to take a long time to execute. Worse, the query could fail.
-
Optimize joins
- When you join two tables, specify the larger table on the left side of join and the smaller table on the right side of the join. Presto distributes the table on the right to worker nodes, and then streams the table on the left to do the join. If the table on the right is smaller, then there is less memory used and the query runs faster.
-
Optimize GROUP BY
- The GROUP BY operator distributes rows based on the GROUP BY columns to worker nodes, which hold the GROUP BY values in memory. As rows are being ingested, the GROUP BY columns are looked up in memory and the values are compared. If the GROUP BY columns match, the values are then aggregated together.
-
Use approximate functions
- For exploring large datasets, a common use case is to find the count of distinct values for a certain column using COUNT(DISTINCT column). An example is looking at the number of unique users hitting a webpage.
-
Only include the columns that you need
- When running your queries, limit the final SELECT statement to only the columns that you need instead of selecting all columns. Trimming the number of columns reduces the amount of data that needs to be processed through the entire query execution pipeline. This especially helps when you are querying tables that have large numbers of columns that are string-based, and when you perform multiple joins or aggregations.
In part-2 of this series, we will discuss a sample architecture to create a data pipeline and the desired file formats.
Link to Data Lakes Part 2
AWS Data Lakes
🔗 Read more about Snowflake here
🔗 Read more about Cassandra here
🔗 Read more about Elasticsearch here
🔗 Read more about Kafka here
🔗 Read more about Spark here
🔗 Read more about Data Lakes here
🔗 Read more about Redshift vs Snowflake here
🔗 Read more about Best Practices on Database Design here